Complex Systems and Network Science
The 21st century has been marked by the unprecedented volume of digital data being increasingly produced on human behavior, biological organisms, economies, and a variety of other complex systems.
Networks delineate the constituent interactions of a broad range of such large-scale complex systems. They provide an essential mathematical representation of socio-economical relations, the human brain, cell metabolism, ecosystems, epidemic spreading, informational infrastructure, transportation systems, and many more.
The structure of these network systems is typically large and heterogeneous, and the interactions they describe are often non-linear, and result in nontrivial emergent behavior and self-organization.
Although network theory offers a wide ranging foundation to untangle such intricate systems, potentially allowing us to predict and control their behavior, the analysis of network data is particularly challenging. Since networks are high-dimensional relational objects, low-order statistics can reveal only very little about them. Conversely, higher-order representations are prone to overfitting, if obtained heuristically, and can easily yield misleading characterizations and statistical illusions.
Our ambition is to render obsolete the reliance on ad hoc heuristics in the field of network data analysis, and transition instead to a mature and robust methodological framework that is derived from fundamental principles and is grounded in solid statistical theory.
Such a framework should be able to extract from data the most appropriate level of complexity that can be justified from statistical evidence, taking into account both epistemic and aleatoric uncertainty, while achieving interpretability, algorithmic efficiency, and versatility.
A central concern of ours is the practical implementation of inductive reasoning and statistical inference to relational data that come from a variety of complex systems in the real world. A lot of what we do is framed by the following instrumental questions:
- How do we prevent overfitting and produce explanations of empirical observations that correctly separate structure from randomness?
- How can we reconstruct dynamical rules and network structures from indirect information on their behavior?
- How do we faithfully model the hierarchical, modular, higher-order, and dynamical structure of network systems?
This line of work was recognized with the Erdős–Rényi Prize from the Network Science Society.
Most of the methods developed in our group are made available as part of the graph-tool library, which is extensively documented. For a practical introduction to many inference and reconstruction algorithms, please refer to the HOWTO.
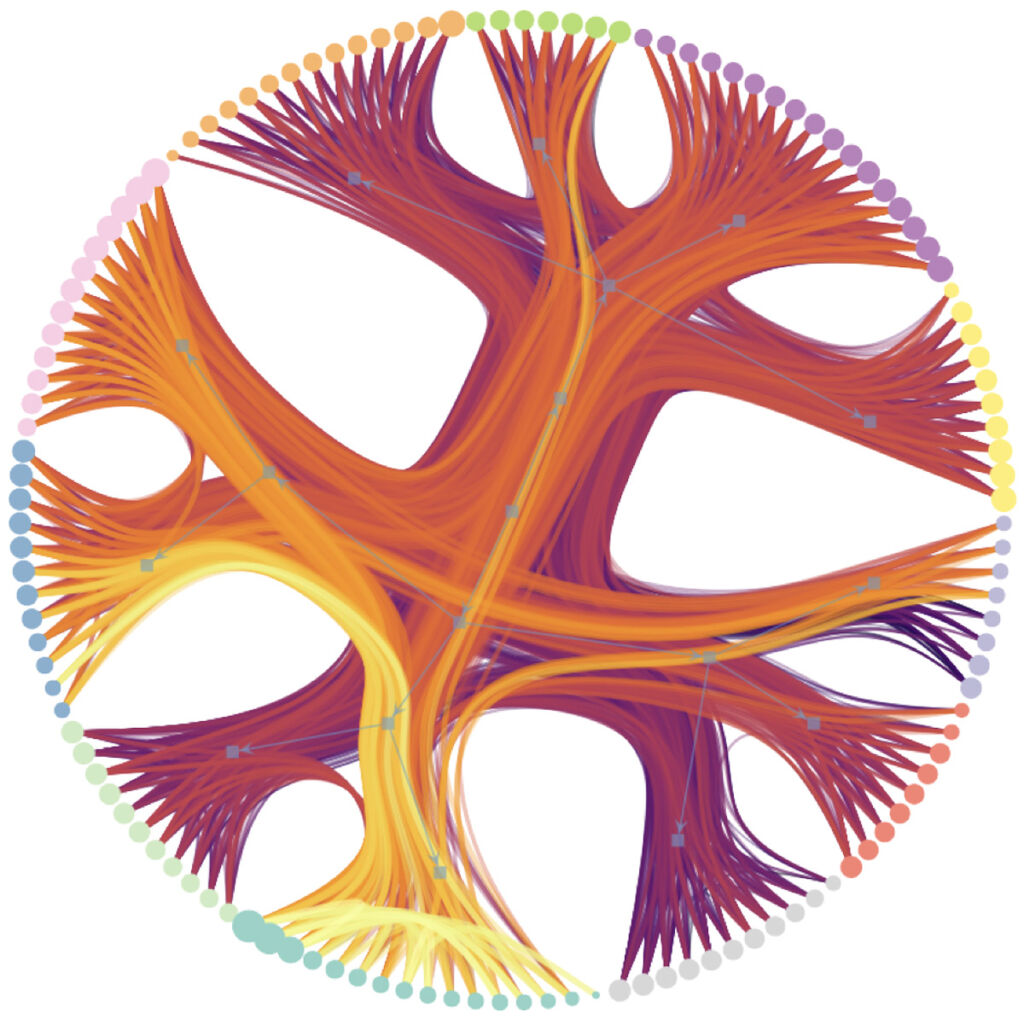