Complex Systems and Network Science
Organisms, ecosystems, climates, information systems, and societies are examples of complex systems: they exhibit rich behavior emerging from a large number of individual components following relatively simple local rules, based on a network of direct interactions.
Complexity science is traditionally devoted to solving the forward problem: Given postulated local rules of interaction, what is the large-scale organization that emerges from them?
Our work at the “Inverse Complexity Lab” focuses on the inverse problem: Given an empirically observed large-scale organization, what are the local rules of interaction that caused it?
With this aim, our group develops mathematical and computational models to explain the structure and function of complex network systems, and the algorithms to reconstruct the structure of these models from available empirical data.
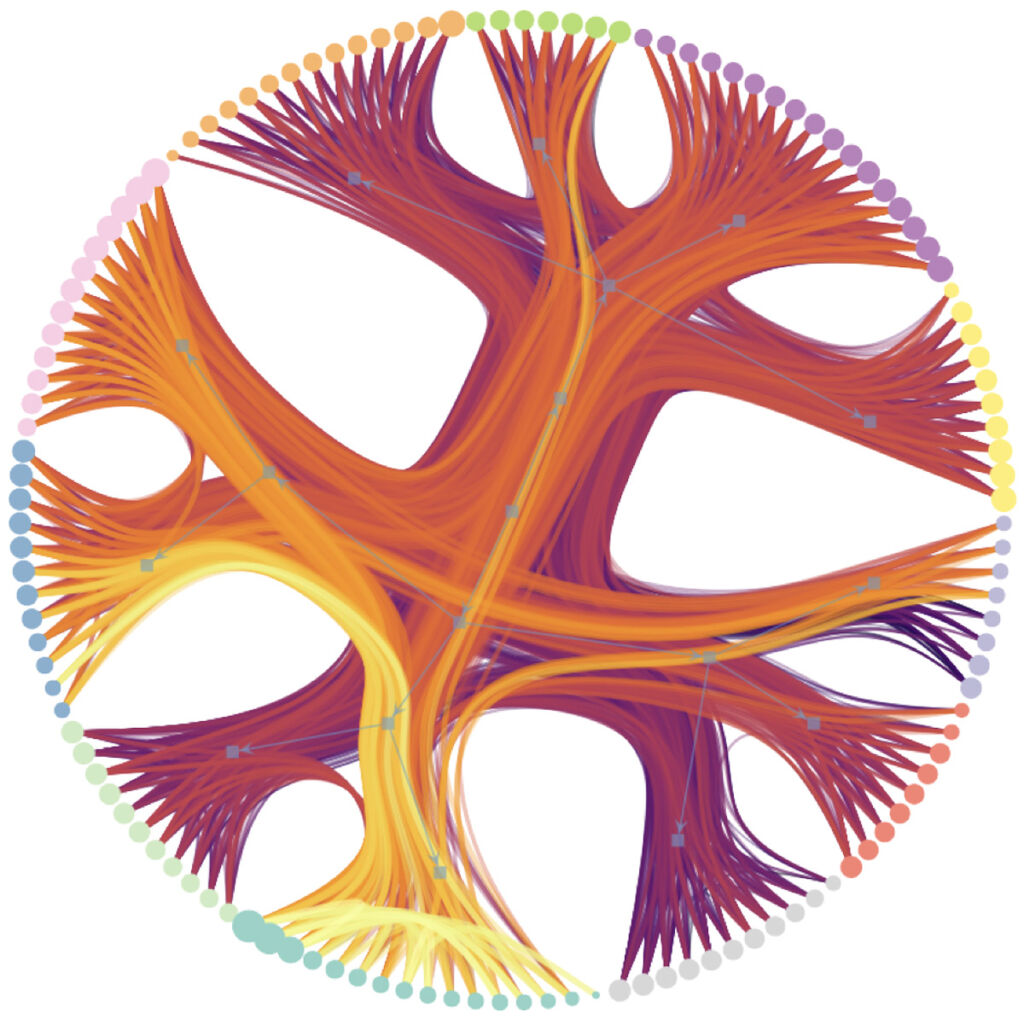